In a recent study published in the journal Nature Cardiovascular Research, researchers used deep learning (DL) assessment of the scarring in the heart to predict arrhythmia-associated sudden death survival.
Study: Arrhythmic sudden death survival prediction using deep learning analysis of scarring in the heart. Image Credit: SquareMotion / Shutterstock
Background
Arrhythmia-related sudden cardiac death (SCDA) is a leading cause of mortality globally. Implantable cardioverter devices (ICDs) efficiently thwart sudden cardiac death (SCD) associated with ventricular arrhythmias. However, existing clinical criteria for ICD candidature, i.e., a left ventricular ejection fraction (LVEF) of less than 30 to 35%, only capture about 20% of all SCDAs. These findings emphasize the crucial need for tailored, precise, and inexpensive arrhythmia risk assessment methods to help alleviate this massive public health and economic hardship.
Moreover, existing evidence suggests that computational models are ineffective as a first-stage screening instrument in a large population. Raw contrast-enhanced, such as late gadolinium enhancement (LGE), cardiac images depicting scar distribution in the DL paradigm that incorporates conventional clinical variables might overcome these constraints and yield precise patient-specific SCDA probability in split seconds.
About the study
In the current retrospective research, the scientists developed a unique DL strategy for individuals with ischemic heart disease to predict SCDA risk. This novel DL technique, named survival study of cardiac arrhythmia risk (SSCAR) methodology, combined the survival analysis and neural networks to estimate individualized survival curves in coronary artery disease from clinical variables and contrast-enhanced cardiac magnetic resonance (CMR) imaging. SSCAR predicted individual patient times to SCDA (TSCDA).
Additionally, the high generalizability and performance of the DL-based learning architecture were assessed using multi-center internal validation data and a separate test set. SSCAR was made up of two neural networks: 1) a three-dimensional (3D) convolutional system utilizing raw unsegmented LGE-CMR images that depict the distribution of scars induced by heart diseases and 2) a densely integrated network that operates on clinical variables.
Top panel (yellow) shows patient data used in this study. SSCAR uses contrast-enhanced (LGE)-CMR images with the left ventricle automatically segmented (left inset) and clinical covariates (right inset; see Methods and Table 1 for a complete list) as inputs to the two sub-networks (left and right pathways). Labels associated with each patient (SCDA events, middle inset, dot-dashed contour)—consisting of the observed times to event and indicators whether the events were SCDA or non-SCDA—are used as targets during training only. LGE-CMR data is taken as input by a 3D convolutional neural network constructed using an encoder-decoder architecture (red panel, left). Clinical covariates are fed to a dense neural network (green panel, right). The sub-networks are trained to estimate two parameters (location μ and scale σ) specific to each patient, which fully characterize the probability distribution of the patient-specific time to SCDA (top blue panel; the time to SCDA is modeled as probabilistic, assumed to follow a log-logistic distribution). During training (dot-dashed arrows and white middle panel), the neural network weights are optimized via a maximum likelihood process, in which a probability distribution is sought (blue double-headed arrow in the middle white panel) to best match the observed survival data (yellow ‘x’s in the middle white panel). Finally, the optimized probability function is used on test LGE-CMR images and covariates to predict patient individualized survival curves (blue bottom panel). Image Credit: Nature Cardiovascular Research (Nat Cardiovasc Res) ISSN 2731-0590 (online)
Results
The authors mentioned that the present DL-projected survival curves provide precise estimates of SCDA for around 10 years and permit the assessment of prediction uncertainty. Findings from an autonomous test set and internal validation data from multiple centers demonstrated concordance indexes of 0.74 and 0.83 and 10-year interconnected Brier scores of 0.14 and 0.12, respectively.
The results indicated that SSCAR was not only a very flexible model capable of capturing intricate non-imaging and imaging feature interrelatedness but also a robust model due to the statistical methodology that governs how these characteristics were merged to suit the survival data. The current framework predicted the TSCDA's whole probability distributions, enabling prediction uncertainties to be patient-specific and based on evidence, giving the model a self-correction mechanism. This method addresses a well-known major problem of neural networks, i.e., excessive confidence in incorrect predictions. The team mentioned that using SSCAR deep neural network learning inside a survival analysis and the resulting precise outputs might constitute a paradigm change in the mode of SCDA risk evaluation.
The present DL method used raw, unsegmented LGE-CMR images as input and automatically finds features that best model and forecast the TSCDA. SSCAR was an SCDA risk prediction paradigm that combined raw imaging with other data sources in the same DL architecture. It used LGE-CMR images and clinical factors in an integrated feature learning method, allowing the various data types to guide the overall survival prototype additively. Standard manually determined imaging characteristics were among the clinical variables employed in SSCAR, prohibiting the CMR neural network from re-discovering the known features and encouraging it to learn new ones. In addition, SSCAR outperformed the state-of-the-art SCDA probability categorization within patients and precisely calibrated SCDA probability.
Conclusions
The study findings revealed that the authors' technique marks a fundamental shift in the strategy of arrhythmia risk analysis, as SSCAR uses the information for directly measuring uncertainty in its projections. The current DL method, in which the input was raw cardiac images, performs better than typical survival models built using clinical variables. This method could revolutionize therapeutic decision-making by providing generalizable and precise estimates of patient-specific arrhythmic death survival probability over time.
Therefore, the researchers are confident that SSCAR could significantly influence clinical decision-making on arrhythmia risk by estimating the TSCDA and a perspective of how specific the model was about each anticipated TSCDA, rather than a simple at-risk or not-at-risk forecast.
- Popescu, D.M., Shade, J.K., Lai, C. et al. Arrhythmic sudden death survival prediction using deep learning analysis of scarring in the heart. Nat Cardiovasc Res 1, 334–343 (2022). DOI: https://doi.org/10.1038/s44161-022-00041-9, https://www.nature.com/articles/s44161-022-00041-9
Posted in: Device / Technology News | Medical Research News | Medical Condition News | Disease/Infection News
Tags: Arrhythmia, Cardiac Arrest, Coronary Artery Disease, Deep Learning, Gadolinium, Heart, Heart Disease, Imaging, Ischemic Heart Disease, Mortality, Public Health, Research, Scar, Sudden Cardiac Death
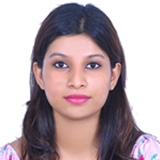
Written by
Shanet Susan Alex
Shanet Susan Alex, a medical writer, based in Kerala, India, is a Doctor of Pharmacy graduate from Kerala University of Health Sciences. Her academic background is in clinical pharmacy and research, and she is passionate about medical writing. Shanet has published papers in the International Journal of Medical Science and Current Research (IJMSCR), the International Journal of Pharmacy (IJP), and the International Journal of Medical Science and Applied Research (IJMSAR). Apart from work, she enjoys listening to music and watching movies.
Source: Read Full Article